Nowadays a big vision of the automotive industry is autonomous driving. Since Google’s introduction of autonomously driving cars, car manufacturers, their suppliers, but also IT companies and the scientific community are excited about the upcoming revolution of transportation. Every transportation-related business will be changed by this new technique and further businesses, which don’t exist yet, will be invented. The biggest advantages of autonomous driving are a higher driving comfort, and assumed the driving systems work reliably, a better driving safety. As soon as drivers won’t have to concentrate their attention on the traffic anymore, they will have spare time during traveling to do other things like surfing in the internet or visiting virtual reality (VR) worlds. I think, that especially these future applications during “be transported” is the driving force for companies like Google to invest millions and billions of dollars into the development of autonomous driving.
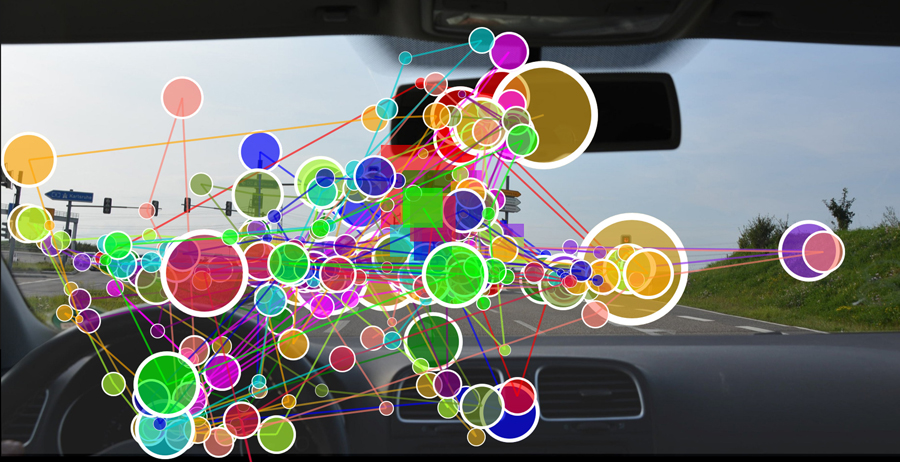
However, there are many issues that have to be resolved until autonomous driving can be fully realized. In my opinion, the most important issue is the driver him- or herself. Since autonomous driving will not be realized in its full functionality immediately, there will be semi-automatic cars for many years on the roads. These semi-automatic cars will have the possibility to drive in some situations for themselves, like for example on the highway. However, in other situations, such as in cities or in complex situations, the driver will still be in charge and has to control the car himself. And this is, were a safe hand over process will be the basic for realizing reliable semi-automatic driving cars.
Imagine a situation like this: You’re driving down a road to a really busy intersection with lots of people in cars and on motorcycles, and even pedestrians. Additionally, there are road signs and traffic lights. Some seconds before your car is reaching this intersection, it decides to hand over control to you. There can be many reasons, why the autonomous driving algorithms decide to do so from no clear sensor data to juridical reasons.
Eye Tracking – A solution for Automatic Driving
The big question is now how to design this hand over process in a way, that will successfully work in almost every driving situation on every location on the world. This is where eye tracking comes into play! A possible solution could look like this: An eye tracking device records eye movements in this situation. Figure 1 shows scan paths of twenty drivers in this situation. Then a specialized computer vision method maps the visual rays with objects detected by car sensors in the environment. By doing so, the embedded systems on the cars can find out what drivers might have perceived in this situation and what they did not see. Based on this information, the car can now support the driver and would warn him or her of things that were not perceived fast enough. I think that this warning system is the missing component for a successful realization of automatic driving.
Almost every car company is performing eye tracking experiments today. The most frequent use case is the optimization of interior’s usability. However, our research shows that an increasing number of engineers also want to use eye tracking for developing automatic driving systems. For that reason, they have to analyze hundreds of driving experiments. The higher the number of test drives is, the better they can understand their drivers. With today methods, the effort for analyzing participants is typically increasing linearly with their number. This means for the car manufacturers, their suppliers and research facilities, very high costs for the analysis of these experiments.
Visual Analytics for Eye Tracking
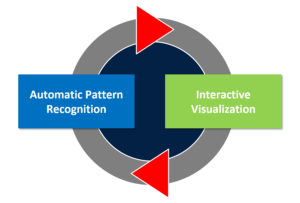
During the last years we intensively have worked on the problem of analyzing large eye tracking data sets. A very promising solution for an efficient analysis of large data sets is the application of the so called Visual Analytics method. This method combines the human ability of finding patterns visually with automatic pattern recognition algorithms and the power of computers, see Figure 2.
In contrast to the psychological approach executing the sequence 1) hypothesis, 2) experiment, and 3) analysis resulting in a significance value, Visual Analytics provides a method for an explorative way of analyzing data. Since Visual Analytics uses computational pattern recognition algorithms, it allows us to analyze eye movements, to find typical scan paths or patterns and detect groups of drivers with a similar eye movement behavior (cf. Figure 3). It also serves as a method to find correlations between eye movements and other information channels. Especially, this feature makes Visual Analytics the perfect technology for the automotive sector, where additional sensor channels such as for the velocity or driving wheel activity are available, see Figure 4.
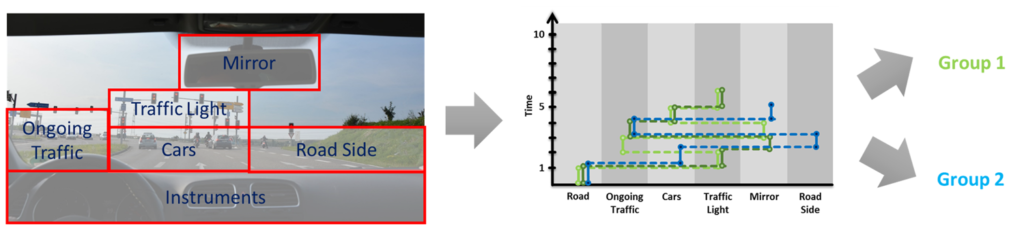
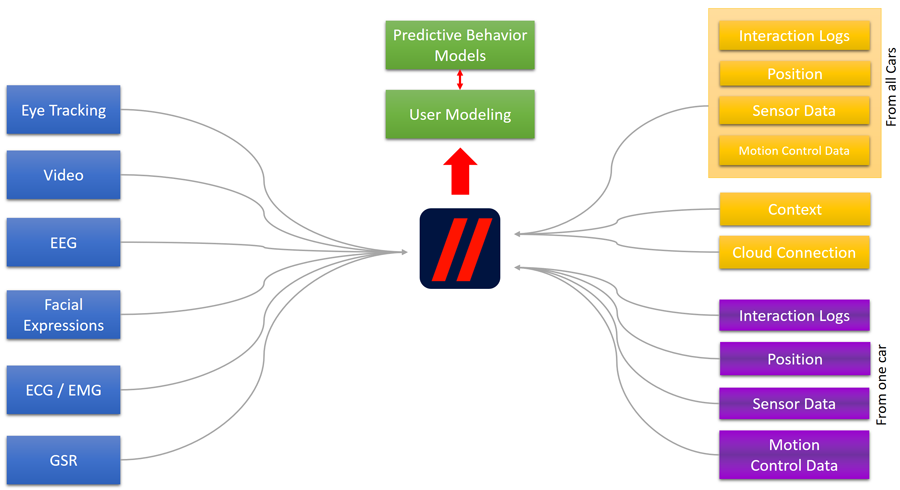
All these data streams can now be integrated into the analysis process. In terms of semi-automatic driving, the car manufacturers can then develop driver models, describing typical driver behaviors in different traffic situations. These models could then serve as the core component for supporting drivers during the hand over process.
The argumentation above seems to be very straight. However, I think, that there exist many open questions and technical challenges between the lines, which have to be discussed in detail. It will be very exciting for us to see how the Visual Analytics method can give its benefit for a successful mastering of these challenges.