After a 4-year journey, I finally finished my PhD with summa cum laude on November 5th, 2024. My thesis is titled “Analysis and Modelling of Visual Attention on Information Visualisations”. For this blog, I summarized the highlights of my thesis for a 5-minute reading.
Understanding and predicting human visual attention have emerged as key research topics in information visualisation research. After all, knowing where users might look provides rich information on how users explore visualisations. However, research on these topics is still severely limited with regard to the scale of datasets and the generalizability of computational visual attention models.
To address these limitations, my thesis aims to collect, understand, and predict human visual attention on information visualisations. My contributions can be divided into three categories: visual attention dataset, analysis, and modelling (see Figure 1). The thesis with five scientific publications progresses through four key stages, which I briefly want to sketch in the following.
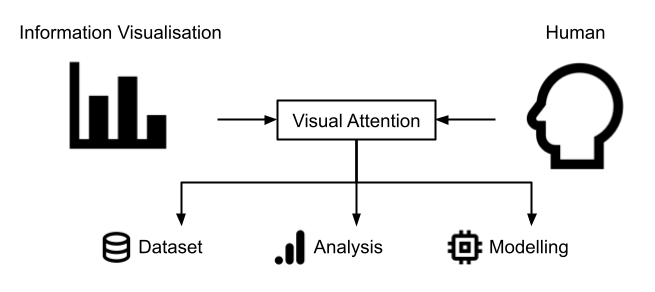
Stage 1: Datasets
My thesis addresses the scarcity of visual attention data in the field by collecting two novel datasets. The SalChartQA dataset contains 6,000 question-driven saliency maps on information visualisations (see Figure 2). Meanwhile, the VisRecall++ dataset contains users’ gaze data from 40 participants with their answers to recallability questions.
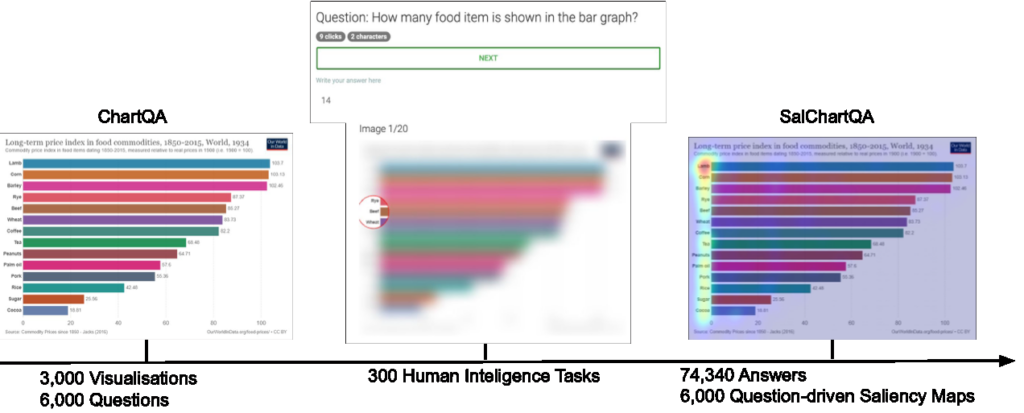
Stage 2: Analysis
Based upon the collected and public visual attention data, the thesis investigates multi-duration saliency of different visualisation elements, attention behaviour under recallability and question-answering task, and proposes two metrics to quantify the impact of gaze uncertainty on AOI-based visual analysis. This work is collaborated with SFB-TRR 161 project B01.
Stage 3: Modelling
Building upon these insights, my thesis proposes two visual attention and scanpath prediction models. VisSalFormer is the first model to predict question-driven saliency, outperforming existing baselines under all saliency metrics (see Figure 3). The Unified Model of Saliency and Scanpaths predicts scanpaths probabilistically, achieving significant improvements in scanpath metrics.
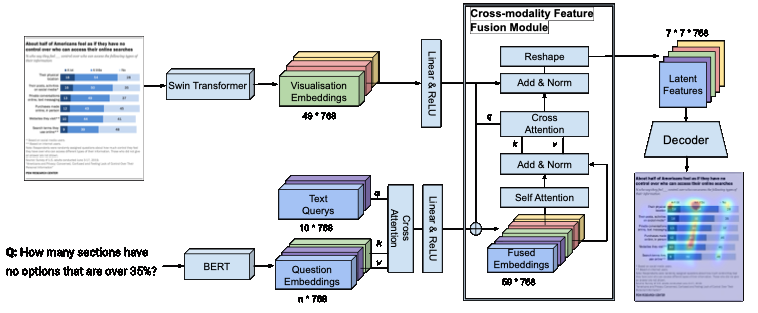
Stage 4: Quantifying visualization recallability
The thesis proposes a question-answering paradigm to quantify visualisation recallability (see Figure 4). It further establishes connections between gaze behaviour and recallability, enabling predictions of recallability from gaze data.
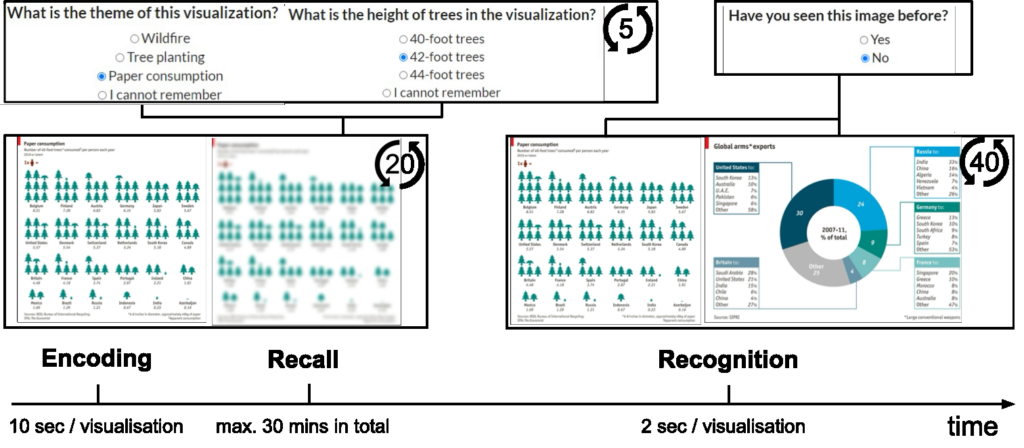
I was fortunate to join the SFB-TRR 161 and the Collaborative Artificial Intelligence (CAI) lab in Stuttgart. I would like to thank my advisor Prof. Andreas Bulling for the continuous support during my PhD, and Prof. Mihai Bâce, Dr. Zhiming Hu, and all other co-authors for their efforts as a part of my thesis work. I also want to thank my family for the all-time love. Finally, I would like to thank the DFG for funding this research within project A07 of the SFB-TRR 161.
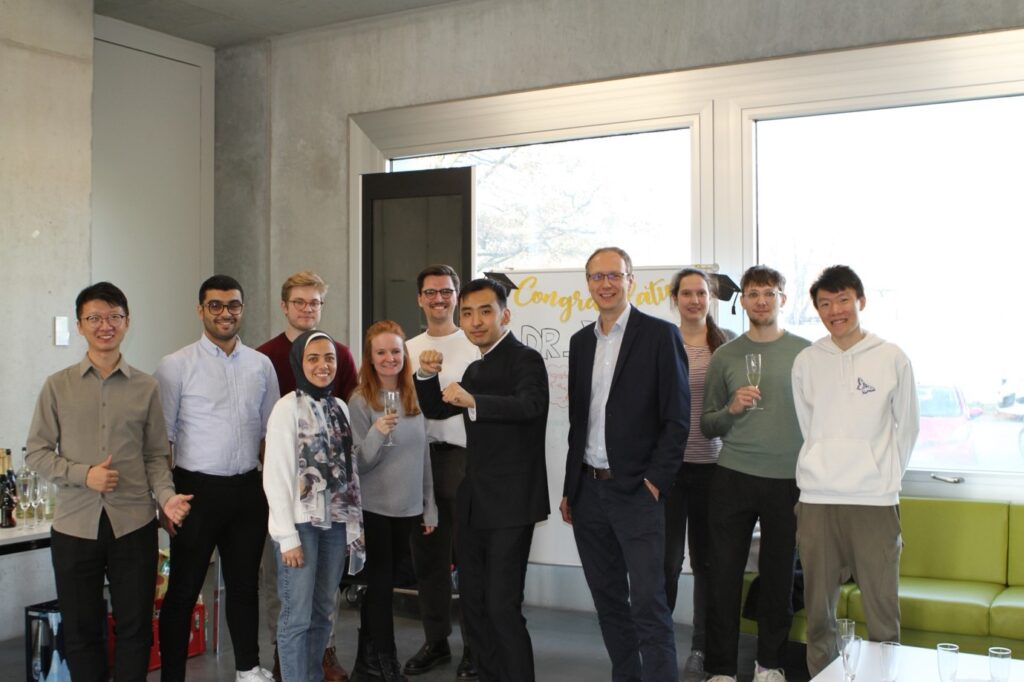