The SFB/Transregio 161‘s Graduate School had the pleasure to attend a workshop on Machine Learning: Dimension Reduction Techniques, which was organized by Prof. Dr. Michael Sedlmair and Dr. Leonel Merino, both from the University of Stuttgart. The workshop was held virtually via Webex due to the ongoing Corona crisis, but was still a great success, thanks to Dr. Michaël Aupetit, Senior Scientist at Qatar Computing Research Institute (QCRI), Hamad Bin Khalifa University, Qatar, sharing his broad knowledge on the topic.
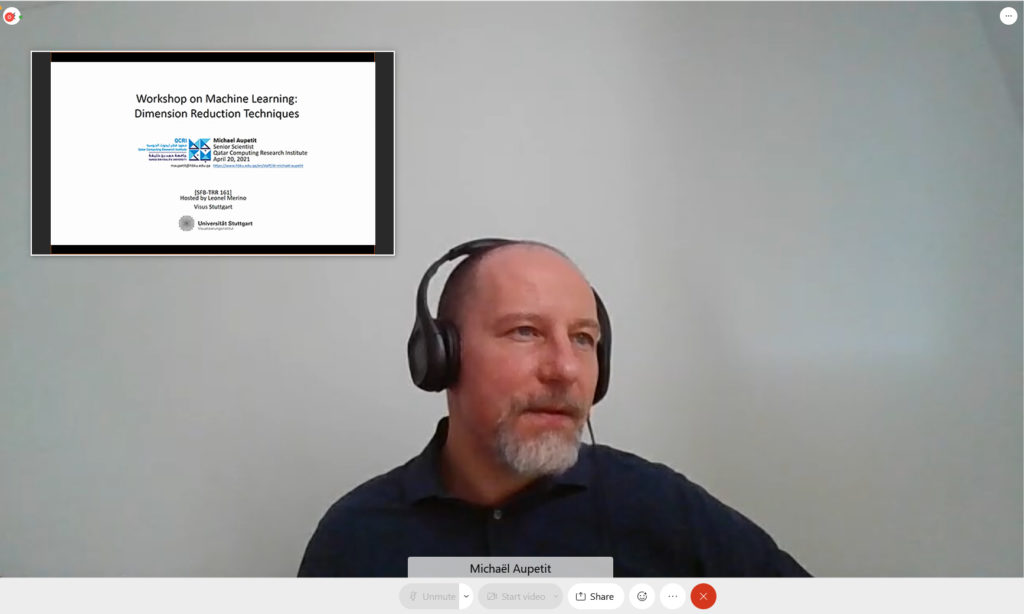
After remembering that one has to click the join-button twice to join a Webex meeting, the workshop started on a funny note two minutes after the scheduled time with a brief introduction of Michaël Aupetit and his work by Michael Sedlmair. Then, after quickly assuring whether the screen sharing was functioning as intended (as has to be usually done in online presentations…), Michaël Aupetit took over and gave an overview of the interesting outline of the ensuing workshop.
Since most of the audience was specialized in Visualization rather than Machine Learning (ML), he decided to give a broader introduction to computational ML techniques for analyzing data with the aim of recognizing data structures via ML (exploratory data analysis). He started by asking how to visualize high-dimensional visual data while preserving similarity based patterns and went on to answer the question by introducing Multi-Dimensional Projections (MDP), going into more detail later on.
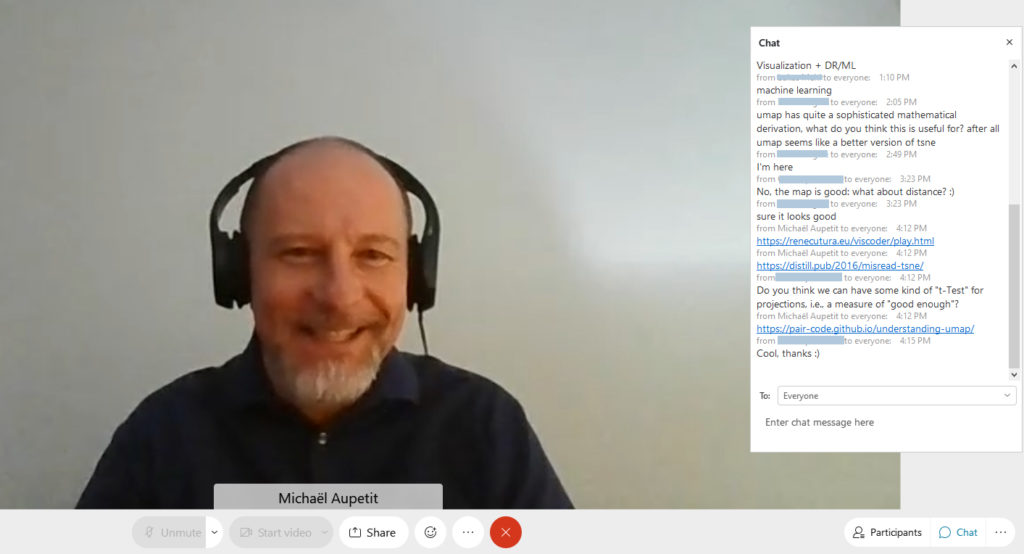
The workshop continued with the presentation of multiple old and new dimension reduction techniques ranging from Principal Component Analysis (PCA), where an eigenvalue decomposition of the covariance matrix of the data is computed, over K-Means and Self-Organizing Maps (SOM), which use K-Means with entangled mean values, on to a comparison between t-distributed Stochastic Neighbor Embedding (t-SNE) and Uniform Manifold Approximation and Projection (UMAP), the latter doing its job faster. In conclusion, the choice of technique is dependent on the data characteristics and the goals to achieve. What type of data and patterns do we have, do we need interpretable axes, interactive parameters and do we account for data labels?
After a short break, Michaël Aupetit went into more detail about MDP and its taxonomy, talking about scalability, steerability and data distortions by dimension reduction and the question on how to limit the impact of distortions at visualization stage. He emphasized the importance of reviewing the data space before disregarding a classification solely on the visuals. Layout Enrichment, that is the act of including information form the data space in the map space, is helpful to analyze distortions correctly.
Future work is to improve the techniques of MDP and Layout Enrichment and to explore MDP to possibly assist ML in regards of explainable Artificial Intelligence.
The workshop was concluded with a demonstration of VisCoDeR, a tool to interactively explore different dimension reduction techniques and their characteristics (links provided below). The tool was developed by Rene Cutura, Stefan Holzer, Michaël Aupetit and Michael Sedlmair.
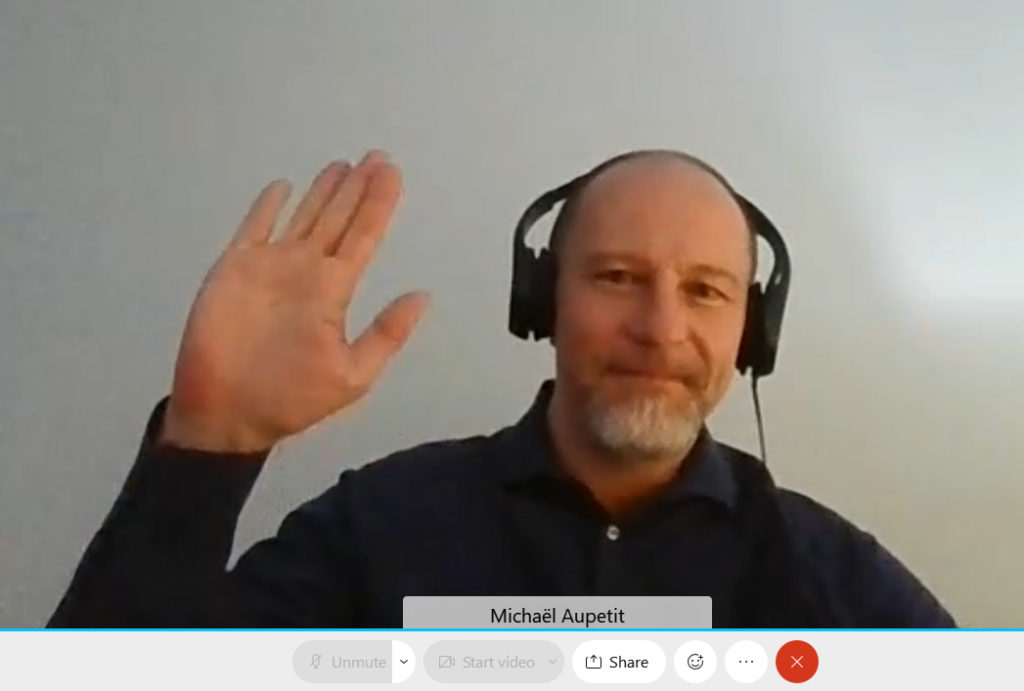
It was a really interesting workshop that might also open up the way for new collaborations for PhD students and Postdocs, which we will hopefully be hearing about in the future.
VisCoDeR:
https://renecutura.eu/viscoder/
https://renecutura.eu/viscoder/explore.html
Thank you Cedric for this great and accurate overview! Thank you to all attendees for the thoughtful discussions.
Best wishes