Digital images have become omnipresent in modern life. The popularity and affordability of handheld imaging devices, especially smartphones, along with the rapid development of social media and social networking services such as Facebook, Flickr, and Instagram have made images a popular and integral part of everyday communication.
With the development of visual media transmission systems, it is becoming increasingly important to improve visual applications in order to meet the quality expectations of end users. For this aim, it is important to evaluate the image quality directly from the user’s perspective, which is known as quality of experience.
The most reliable way of assessing the quality of an image is a subjective evaluation. However, it is expensive, time-consuming, and cannot be used for large-scale and automatic image quality assessment. To overcome these limitations, objective image quality assessment methods were proposed. The aim is to supply quality metrics that can predict perceived image quality automatically and accurately.
Technical Quality Assessment
Recently some image quality assessment methods including our BIQWS metric were proposed for assessing the technical quality such as noise, compression artifacts, blur, etc. by modeling the statistics of natural scenes. The assumption is that the natural scene statistics are changed by distortions. These changes are estimated to extract technical quality aware features. Then the model is trained with data labeled by humans.
However, these methods neglect the influence of image content and aesthetics on the perceptual quality judgment. The following figure shows some images from TID2013 database, their corresponding predicted quality scores by our BIQWS method, and their subjective scores (inside the parenthesis).
Aesthetics Aware Quality Assessment
To fill this gap, we proposed a model which integrates features for assessing both visible distortion and aesthetics. In the following figure, two pristine high-quality images from TID2013 database were distorted by the same distortion with the same severity but their subjective scores are very different. These scores show that the aesthetics and semantic level characteristics of the images strongly affect the perceptual quality judgment.
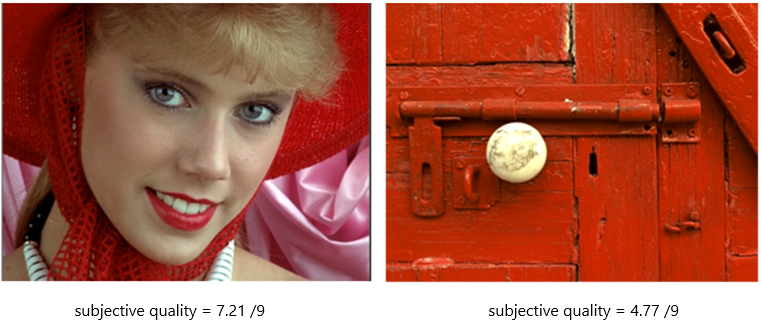
We studied 32 image aesthetics measures were commonly used in the fields of evolutionary art and aesthetic quality assessment and modified some of them to get better performance. The goal of evolutionary art is to generate an attractive image, and the aesthetic prediction methods categorize images as low or high aesthetic quality.
Then a feature section algorithm was used to select the efficient set of these aesthetic measures which achieve the highest correlation with the subjective opinion on the large-scale database for Aesthetic Visual Analysis (AVA). Eleven features were selected including features related to image hue composition, colorfulness, fractal dimension, global contract, etc.
Then, the quality-aware features of the distortion aware image quality method were augmented by the aesthetic features. A machine learning approach is used to map the feature space to the subjective scores.
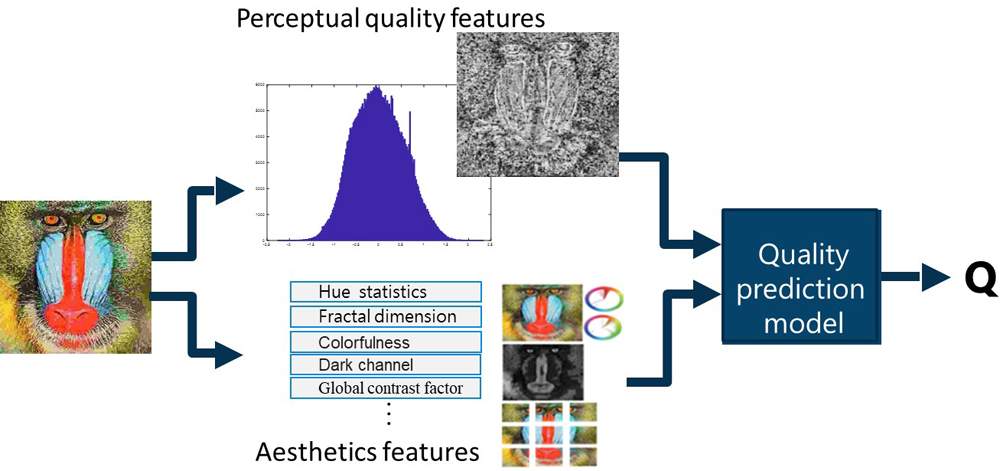
Our proposed model can score input images with high correlation to human perception. It can be used for monitoring image quality degradations and benchmarking image processing systems. Also, they help to optimize many image processing algorithms and systems such as image encoding methods. Following figure shows the predicted scores and their corresponding subjective score (inside parentheses) for some image from KonIQ-10k natural image database.